Personalized Nutrition: The Intersection of Big Data and Diet
How Artificial Intelligence and Informatics can shape diets according to each individuals genetic information.
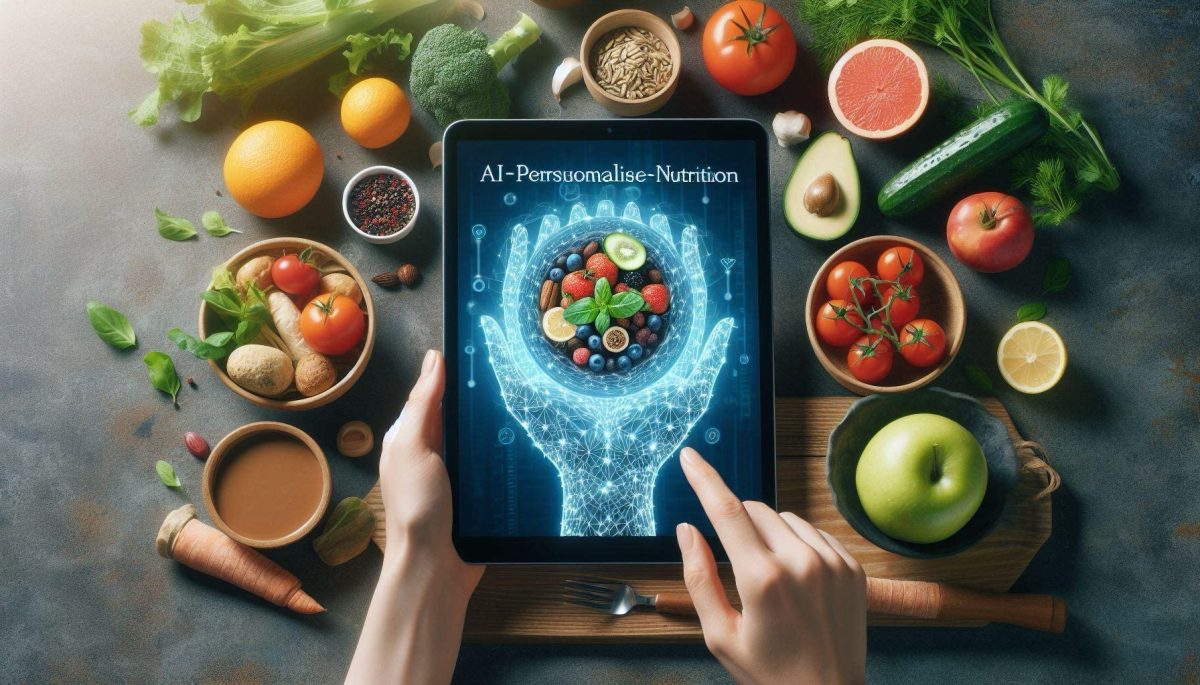
Introduction
Nutritional science has traditionally relied on broad dietary guidelines that aim to serve the general population. However, increasing evidence suggests that interindividual variability in metabolism, genetic predisposition, and microbiome composition plays a significant role in how individuals respond to specific dietary patterns (Zeevi et al., 2015). The concept of personalized nutrition seeks to address these variations by leveraging genetic information, metabolic biomarkers, microbiome sequencing, and artificial intelligence (AI) to develop customized dietary interventions.
Recent technological advances, including continuous glucose monitoring, machine learning algorithms, and high-throughput sequencing, have enabled a more precise approach to dietary recommendations. This article explores the key scientific principles underlying personalized nutrition, the methodologies used to develop individualized diet plans, and the implications for health and disease prevention.
Genetic Variability and Nutrigenomics
The field of nutrigenomics investigates how genetic variations influence nutrient metabolism, dietary preferences, and disease risk. Single nucleotide polymorphisms (SNPs), which are small genetic variations at a single DNA base pair, can significantly impact an individual's ability to metabolize certain nutrients (Ordovas et al., 2018).
For example, the LCT gene encodes lactase, an enzyme responsible for lactose digestion. Individuals carrying a non-functional allele of the LCT gene experience lactose intolerance due to reduced lactase production. Similarly, polymorphisms in the FTO gene have been linked to differences in fat metabolism and obesity risk, influencing how individuals respond to high-fat diets (Loos & Yeo, 2022).
Research has demonstrated that tailoring dietary interventions based on genetic markers can improve metabolic outcomes. A randomized controlled trial by Livingstone et al. (2021) showed that individuals who followed a genetically guided diet experienced greater reductions in body weight and blood lipid levels compared to those adhering to standard dietary guidelines.
The Gut Microbiome and Metabolic Responses
The gut microbiome—comprising trillions of bacteria, fungi, and viruses—plays a crucial role in digestion, nutrient absorption, and immune function. Recent studies have demonstrated that microbiome composition significantly affects metabolic responses to food.
Zeevi et al. (2015) conducted a large-scale study analyzing postprandial glucose responses in over 800 individuals. Their findings revealed that glycemic responses to identical meals varied widely among participants and were strongly correlated with gut microbiota composition. The study highlighted that microbial diversity and the presence of specific bacterial strains (e.g., Akkermansia muciniphila) influenced glucose metabolism and insulin sensitivity.
Personalized nutrition interventions targeting microbiome composition have shown promising results. A study by Wang et al. (2023) demonstrated that dietary modifications designed to promote beneficial gut bacteria led to significant improvements in glucose homeostasis and lipid metabolism in prediabetic individuals.
Wearable Technology and Real-Time Nutritional Feedback
Advancements in continuous glucose monitoring (CGM) and wearable biosensors have enabled real-time tracking of dietary effects on metabolic health. These devices measure blood glucose fluctuations, heart rate variability, and other physiological parameters to assess how specific foods impact individual metabolism.
A study by Jarvis et al. (2023) demonstrated that CGM-guided dietary interventions resulted in a 27% improvement in glucose control compared to conventional dietary recommendations. By integrating machine learning algorithms, AI-driven applications such as ZOE and DayTwo analyze CGM data alongside microbiome and genetic profiles to generate personalized meal plans.
Moreover, metabolomic profiling—analyzing blood biomarkers to assess real-time metabolic responses—has further refined personalized nutrition approaches. Recent research has shown that incorporating metabolomics data into dietary interventions enhances precision, leading to better metabolic regulation and reduced inflammation (Johnson et al., 2021).
Artificial Intelligence in Personalized Nutrition
Machine learning and AI-driven models are at the forefront of personalized nutrition research. These technologies integrate vast datasets—including genetic markers, microbiome profiles, real-time glucose data, and dietary intake—to develop predictive models for dietary responses.
Deep learning algorithms have been trained on large cohorts to identify patterns in metabolic responses. For instance, a study Segal et al. (2021) utilized AI to predict glycemic responses to meals with 82% accuracy, outperforming traditional carbohydrate-counting methods. These predictive models allow for real-time dietary adjustments to prevent hyperglycemic or hypoglycemic episodes in individuals with diabetes.
Furthermore, AI-powered chatbots and virtual dietitians provide real-time dietary recommendations, improving adherence to personalized diet plans. Research by Patel et al. (2024) found that AI-driven diet coaching increased compliance with personalized nutrition interventions by 35% compared to self-guided approaches.
Clinical Applications and Health Benefits
Personalized nutrition has demonstrated significant potential in preventing and managing chronic diseases:
- Diabetes Management: AI-driven dietary interventions reduce postprandial glucose spikes and improve insulin sensitivity.
- Cardiovascular Health: Nutrigenomics-based diets lower LDL cholesterol and improve lipid profiles in genetically predisposed individuals.
- Weight Management: Personalized diets based on genetic and metabolic data are more effective for long-term weight loss than generic calorie-restriction approaches.
- Gastrointestinal Disorders: Microbiome-targeted nutrition plans alleviate symptoms of irritable bowel syndrome (IBS) and inflammatory bowel disease (IBD).
Challenges and Ethical Considerations
Despite its potential, personalized nutrition faces several challenges:
- Data Privacy and Security: The collection of genetic and metabolic data raises ethical concerns regarding privacy and consent.
- Cost and Accessibility: Advanced genetic and microbiome testing remains expensive and is not widely available.
- Regulatory Uncertainty: Standardized guidelines for AI-driven dietary recommendations are lacking, leading to variability in service quality.
- Scientific Validation: While promising, large-scale, long-term clinical trials are needed to validate personalized nutrition approaches.
Conclusion and Future Directions
Personalized nutrition represents a paradigm shift in dietary science, moving away from one-size-fits-all recommendations toward individualized dietary interventions based on genetics, microbiome composition, and AI-driven data analysis. While challenges such as cost, accessibility, and ethical considerations remain, ongoing advancements in biotechnology and computational modeling are expected to refine and expand personalized nutrition applications.
Future research should focus on standardizing protocols, conducting large-scale trials, and integrating blockchain technology to ensure secure data management. As the field continues to evolve, personalized nutrition has the potential to become a cornerstone of precision medicine, improving health outcomes on an individualized basis.